Meaningful Metrics and Scales of Measurement
- Jim Shupe
- Apr 17, 2023
- 5 min read
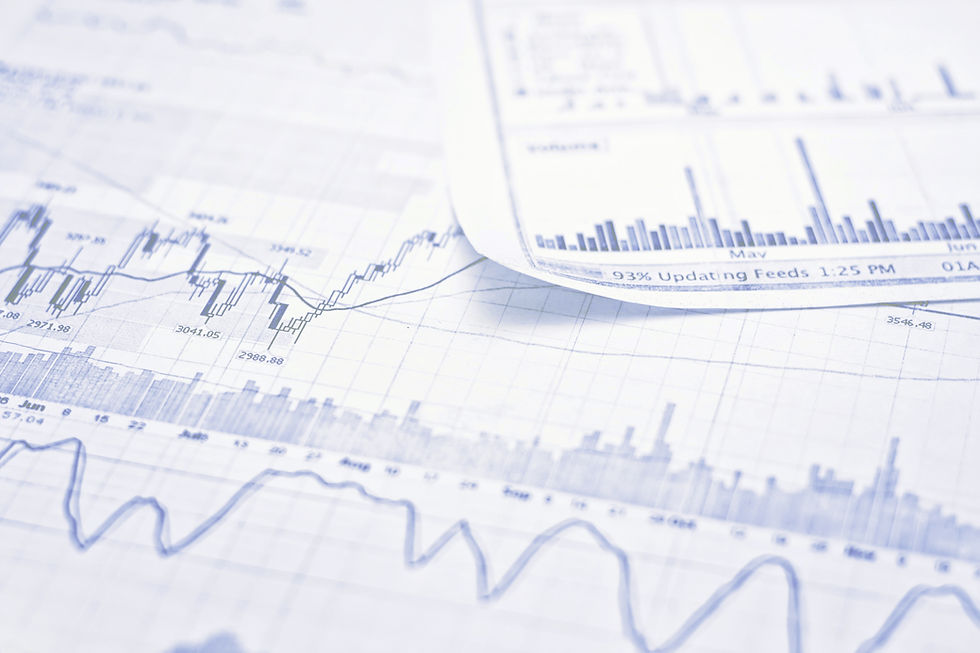
When planned properly, your Key Results will have metrics built into them from the beginning. Not all metrics are equally good, however. The important thing is to make sure that what you are measuring is a strong indication of how well you are actually doing. Keep them focused on the result. Sometimes people measure things that are interesting but not important to the result. When the metrics become the goal, it can cause unwanted results. The Key Result must remain anchored on the Objective. The metrics just tell you how close you are getting.
Here are some examples of metrics gone wrong and how they could be changed to make them better.
One apocryphal story describes a Soviet shoe factory that was told to increase production by a certain amount. They didn’t have enough leather to make all the full-sized shoes needed to meet the new target, so they made children’s shoes instead. This led to an oversupply of kids’ shoes and intensified the shortage among adults.
Improved metric: set a target for the number of shoes per type by size. They should then determine if there are any issues with meeting those targets and decide whether to prioritize production, accept what is possible with existing resources, or get additional resources to meet the target.
During British Occupation, there was concern about the number of deaths from venomous snakes. Local authorities offered a reward for anyone who brought in a dead one. This led to people raising venomous snakes secretly in order to kill them and bring them in for the reward.
Improved metric: set a target to reduce the number of deaths from venomous snakes per year. Care would need to be taken to avoid undercounting deaths by simply having them classified as another cause. This could, however, encourage citizens to look into multiple methods to reduce the number of deaths through education programs to keep people away from the snakes, thinning the population through hunting, or improved medical procedures to keep people who are bitten from dying.
Viet Nam experienced a similar problem with an overpopulation of rats during France's colonial period. They offered bounties on rat tails, thinking that was evidence that the wild population would be reduced. In practice, the rats were bred in captivity for their tails. Once the reward was ended, the rats were released back into the city.
Improved metric: set a target for reduced rat population in specific buildings, reduced grain losses to rat theft, and less rat-related illness. The problem is that the reward was tied to a heuristic, a stand-in value for what they really wanted to measure.
Scales of Measurement
When collecting metrics for a Key Result, you must strike a balance between how precise the measurement is versus how complex it is to collect. According to Adi Bhat, there are four scales of measurement precision, also called levels of measurement. They are in the table below.

Nominal data can be divided into different groups. An example of this would be Yes/ No characteristics such as “Did the student sign the Code of Conduct form?”. Further examples are car brands, country of residence, and favorite sports team.
Ordinal data have an order to them, but the magnitude separating them is unclear. Let’s suppose you wanted to rank the top 5 rivers in the world by volume. From greatest to least they would be the Amazon, Congo, Ganges, Orinoco, and Rio Negro rivers. This does not reveal that if you add together the volume of the second through fifth rivers, the Amazon would still be far larger. In fact, the Amazon’s volume is greater than 5 times the Congo, the second river on the list! These comparisons are not obvious in an ordinal scale where we simply list them from greatest to least.
Interval data allow you to determine the difference between data points using a numeric scale. Since these data points are arranged by numeric value, they naturally have an order to them as with ordinal data, but we can now also measure the distance between any two values. On a scale from 1 to 10, things that score as an 8 will always be greater than things that score a 4. An 8 is also 4 levels higher than a 4. But this does not mean that a meal that scored an 8 out of 10 is twice as good as a meal that scored a 4. This is because the zero level is arbitrary, which is fixed in our final category.
Ratio data have all the characteristics of the previous three and have a natural zero point. In the Top 5 Rivers example, it is possible to measure water flow. Anything scoring a zero would not qualify as a river, of course, but because the volume metric starts at zero and goes up in equal intervals from there, we can now say with certainty that the Amazon is five times as great as the Congo.
Measuring Experiences and Opinions
Sometimes you’re looking to measure something that’s less concrete, less countable. These are called qualitative metrics because they focus on the qualities of a thing or the opinion of others rather than physical, easily counted things. There are a couple of ways to handle this
The first method should be familiar to most, the survey. Asking your target audience how they rate their satisfaction with something on a scale of 1 to 10, as an example, converts the qualitative aspects into easily measured and counted values.
The second method is to define a proxy metric, or a measurable stand-in value that you can observe. An example of this is A-B testing, which Google famously put to great effect in building their search engine dominance 4. In A-B testing, you choose one thing you want to measure. In Google’s case, they found that the link color for ads returned on the search site was a different shade of blue than the one used in Gmail ads. They could have simply chosen one and moved on. Instead, they decided to see whether the color of the links they used for ads had any effect on whether people clicked on them. They chose the current default color to use as a baseline and served that to 99% of their queries. The other 1% would get the alternate color. After a set period, they would end that test and do another with a new color as the 1% alternate until the list of options was complete. In all, they tried 41 different shades of blue. The color rated highest for number of clicks was the winner. This is how they determined the color scheme for ad links we are all familiar with today, and it got them an additional $200 million a year in revenue.
Summary
Make sure your metrics don’t become the goal. Adjust metrics you find that reward the wrong behavior.
Use the scale of measurement that allows you to analyze the data to the level of precision you need, while introducing the least complexity to implement and measure.
Nominal – Can be Categorized (yes/no, country of residence)
Ordinal – Can be ordered (top 5 rivers in the world by volume)
Interval – Can have distance between values measured (a five-star restaurant is better than a four-star one)
Ratio – Can be used to compare magnitude between values due to the scale having a natural zero point. (the Amazon is more than five times as great as the Congo in volume.)
Measure qualitative values using surveys or A-B testing
With A-B testing, choose two values. One is a baseline and presented to 99% of your interactions with the target audience. The remaining 1% get the option under test.Measure whether either gets more of the desired result like link clicks, sales, or referrals. The quality getting the most of the measurable value is the winner